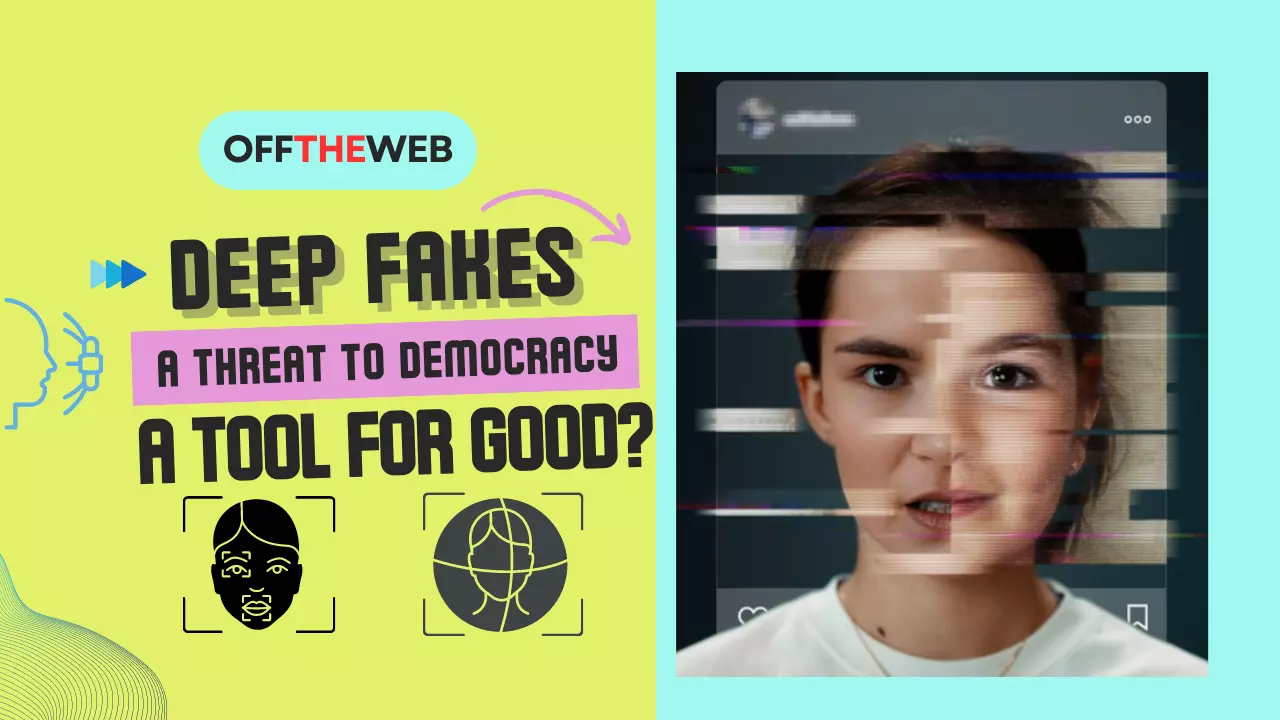
Index
Introduction
Deep Fakes: A Threat to Democracy or a Tool for Good?
In an age where technology has boundlessly blurred the lines between reality and fiction, the emergence of Deep Fakes has sparked both fascination and fear. This cutting-edge AI-driven technique has the astonishing ability to convincingly manipulate visual and audio content, bringing forth an era where distinguishing truth from fiction becomes increasingly challenging. Deep Fakes, once confined to the realms of science fiction, now stand at the precipice of shaping the very foundation of our societies.
On one hand, the potential applications of Deep Fakes for good seem limitless. From revolutionising the entertainment industry with mesmerising visual effects to transforming medical simulations and enhancing accessibility, this technology carries the promise of unlocking innovative possibilities across various fields. However, as the boundaries between authenticity and deception blur, it also poses an alarming threat to the very essence of democracy.
Are Deep Fakes merely a marvel of technological advancement, or do they harbour the potential to disrupt and corrode the democratic principles we hold dear? To answer this pressing question, we shall navigate through the realms of its positive contributions and insidious implications. Moreover, we will examine the challenges that lie ahead in combating the malicious use of Deep Fakes and the imperative need for regulatory measures.
In this article, we will explore the fascinating world of Deep Fakes, questioning their role as either a transformative tool for progress or a menacing harbinger of disinformation and deception. As we delve into the capabilities and limitations of this artificial intelligence phenomenon, we confront the ethical and societal dilemmas that surround its existence.
Deep Fakes and Their Growing Popularity
Deep Fakes refer to the sophisticated and deceptive digital manipulations of visual and audio content using artificial intelligence, specifically deep learning algorithms and neural networks. These AI-driven techniques allow for the creation of highly realistic and often indistinguishable fake media, such as videos, images, and audio, featuring individuals saying or doing things they never actually did.
The term "Deep Fakes" is a blend of "deep learning" (referring to the AI technique used) and "fake." Deep learning is a subset of machine learning that involves training deep neural networks on vast amounts of data, enabling them to learn patterns and create representations of data with exceptional accuracy.
Deep Fakes work by training a neural network on extensive datasets of real video and audio recordings of a specific person. The model learns to understand the facial expressions, speech patterns, and other unique characteristics of that person. Once the model is trained, it can be used to generate new content that convincingly mimics the targeted individual's appearance and voice.
The growing prevalence of Deep Fakes is a cause for concern. Initially, these manipulations were mainly confined to well-funded research labs and studios with extensive computing resources. However, advancements in AI technology, the availability of powerful hardware, and open-source machine learning frameworks have democratised the creation of Deep Fakes. As a result, the tools and knowledge required to generate Deep Fakes have become more accessible to a wider audience, including individuals with malicious intent.
The increasing prevalence of Deep Fakes raises several critical issues:
- Misinformation and Disinformation: Deep Fakes can be used to create fake news and false narratives, making it challenging for individuals to discern between genuine and manipulated content.
- Political Implications: Political figures can be targeted with Deep Fakes, leading to the spread of misinformation, damage to reputations, and manipulation of public opinion.
- Erosion of Trust: As Deep Fakes become more convincing, people may become increasingly sceptical of audio and video evidence, leading to a decline in trust in media and institutions.
- Privacy Concerns: The ability to create realistic fake content raises concerns about privacy violations, as individuals may find themselves featured in manipulated videos or images without their consent.
- Security Threats: Deep Fakes can be used for social engineering, fraud, and blackmail, as individuals may be coerced or tricked into believing fake content is genuine.
The increasing prevalence of Deep Fakes has prompted researchers, tech companies, and policymakers to address the potential risks and develop ways to detect and combat this form of digital deception.
Understanding Deep Fakes
Deep Fakes are created using a combination of artificial intelligence techniques, primarily deep learning algorithms and neural networks. The process involves training a model on a large dataset of real data and then using that model to generate fake content.
- Data Collection: The first step in creating a Deep Fake is to gather a substantial dataset of real media featuring the target person. This dataset typically includes videos, images, and audio recordings of the individual from various angles, facial expressions, and speech patterns. The larger and more diverse the dataset, the better the quality of the resulting Deep Fake.
- Preprocessing: The collected data is preprocessed to extract essential features, such as facial landmarks, voice characteristics, and other relevant information. The goal is to create a representation of the target person that the deep learning model can understand and learn from.
- Training the Deep Learning Model: The heart of creating Deep Fake lies in training a deep learning model, usually a type of neural network, on the collected dataset. The model used is often a Generative Adversarial Network (GAN) or a Variational Autoencoder (VAE). GANs consist of two neural networks – a generator and a discriminator – which compete against each other during training to create increasingly realistic content.
- Generator Network: The generator network is the core component responsible for producing the Deep Fake. During training, it learns to generate content that closely resembles the real data in the training set. It takes random input (often called "latent vectors") and learns to transform them into realistic images, videos, or audio.
- Discriminator Network: The discriminator network, on the other hand, learns to distinguish between real and fake content. Its role is to identify whether the content produced by the generator is genuine or a Deep Fake. Both networks are in a continuous feedback loop, with the generator aiming to produce content that can deceive the discriminator, and the discriminator getting better at detecting fake content.
- Fine-Tuning: Training the deep learning model can be a computationally intensive process, often requiring specialised hardware. Fine-tuning the model involves iterating over the training process, adjusting parameters, and optimising until the desired level of realism is achieved.
- Generating Deep Fakes: Once the deep learning model is adequately trained, it can be used to generate Deep Fakes. To create a Deep Fake video, for instance, the model takes a real video of the target person as input, processes it through the generator network, and produces a modified video that appears to be authentic but contains manipulated facial expressions or speech.
Benefits of Deep Fakes
Deep Fakes, despite their potential negative implications, also have several positive and beneficial uses in various fields.
- Entertainment and Visual Effects: Deep Fakes can revolutionise the entertainment industry by creating hyper-realistic visual effects. They can bring back deceased actors to reprise roles, recreate historical events, and enhance the overall cinematic experience.
- Dubbing and Localization: Deep Fakes can be used to dub movies and TV shows, making them accessible to a global audience in their native languages. This can significantly reduce the time and cost involved in traditional dubbing processes.
- Education and Training: Deep Fakes can be employed to create interactive and engaging educational content. For example, historical figures can "come to life" in history lessons, or language learners can practise conversations with virtual native speakers.
- Medical Simulations: Deep Fakes can be utilised in medical training and simulations. Doctors and medical students can practise complex procedures on virtual patients, offering a safe and controlled environment for learning.
- Accessibility: Deep Fakes can enhance accessibility for individuals with disabilities. For example, sign language interpreters can be generated automatically for online videos, making them more inclusive for the hearing-impaired.
- Film Restoration: Deep Fakes can help restore old and damaged films, enhancing their visual quality and preserving cultural heritage.
- Virtual Avatars and Assistants: Deep Fakes can be used to create realistic virtual avatars or digital assistants that closely resemble real individuals, making interactions with technology more human-like and relatable.
- Character Animation and Gaming: In the gaming industry, Deep Fakes can improve character animations by transferring the facial expressions and emotions of real actors onto virtual characters.
- Architectural Visualisation: Deep Fakes can aid in creating lifelike visualisations of architectural designs, providing clients and stakeholders with a realistic preview of the final project.
- Art and Creativity: Deep Fakes can be utilised as an innovative medium for artistic expression, enabling artists to explore new forms of digital art and creativity.
It is essential to recognize that while these positive uses hold tremendous potential, vigilance and responsible implementation are crucial. Ensuring that Deep Fakes are used ethically and for the betterment of society will help maximise their positive impact while mitigating the risks associated with their misuse. Additionally, the ongoing development of regulations and detection technologies can help maintain a balance between the beneficial and harmful aspects of Deep Fakes.
The Dark Side of Deep Fakes
Deep Fakes present several negative implications and potential risks, which have raised concerns among experts, policymakers, and the general public.
- Misinformation and Fake News: Deep Fakes can be used to create highly convincing fake videos and audio recordings, leading to the spread of misinformation. These manipulated media can be used to generate false narratives, deceive the public, and undermine trust in reliable sources of information.
- Political Manipulation: Deep Fakes can be exploited for political purposes, such as creating fake speeches or interviews of politicians. This can be used to manipulate public opinion, sow discord, and damage the reputation of political figures.
- Identity Theft and Harassment: Individuals can become victims of identity theft when their likeness is used in Deep Fakes without their consent. This can lead to harassment, cyberbullying, and reputational damage.
- Discrediting Authentic Evidence: The existence of Deep Fakes raises doubts about the authenticity of real audio and video evidence. In legal contexts, this can undermine the credibility of genuine recordings and hinder the pursuit of justice.
- Privacy Concerns: The technology behind Deep Fakes can infringe on an individual's privacy, as anyone with access to sufficient personal data and media can create fake content with malicious intent.
- Social Engineering and Fraud: Deep Fakes can be used for social engineering attacks, tricking individuals into believing fake content is genuine. This can lead to various forms of fraud and financial losses.
- Erosion of Trust: The widespread use of Deep Fakes can erode public trust in media, institutions, and digital content, making it increasingly challenging to discern genuine information from manipulated content.
- Spread of Hate Speech and Extremism: Deep Fakes can be used to create inflammatory content, including hate speech and extremist propaganda, which may further polarise society.
- Cybersecurity Threats: The creation and dissemination of Deep Fakes can open up new vectors for cyberattacks, such as spreading malware disguised as fake media.
- Challenges in Detection and Regulation: Identifying Deep Fakes and distinguishing them from genuine content is a significant challenge. The rapid evolution of Deep Fake technology makes it difficult for detection methods to keep up. Moreover, creating effective regulations that balance freedom of expression with the need to prevent malicious use is a complex task.
Addressing the negative side of Deep Fakes requires collaborative efforts from various stakeholders, including tech companies, policymakers, researchers, and the public. Developing robust detection technologies, promoting media literacy, and implementing responsible use guidelines can help mitigate the potential risks associated with Deep Fakes.
The Need for Regulating the Use of Deep Fakes
Ethical concerns surrounding the use of Deep Fakes stem from their potential to deceive, manipulate, and harm individuals, society, and the fabric of truth itself. Regulation is essential to control the malicious use of Deep Fakes and protect individuals and society from their negative consequences.
- Protecting Individuals: Regulations can provide legal safeguards to protect individuals from the non-consensual creation and distribution of Deep Fakes that could harm their reputation, privacy, and well-being.
- Combating Misinformation: Regulations can help combat the spread of malicious Deep Fakes that aim to deceive the public and manipulate information for political or harmful purposes.
- Ensuring Accountability: Regulations can hold creators and distributors of harmful Deep Fakes accountable for their actions, deterring malicious intent and reducing the likelihood of misuse.
- Preserving Trust in Media: By setting standards and guidelines for identifying and labelling Deep Fakes, regulations can help preserve trust in authentic media sources.
- Promoting Ethical Use: Clear regulations can encourage responsible and ethical use of Deep Fakes, promoting creativity, innovation, and positive applications of synthetic media.
- Empowering Detection Efforts: Regulations can incentivize the development of better detection technologies, making it easier to identify and counteract malicious Deep Fakes.
- Balancing Freedom of Expression: Regulations can strike a balance between protecting against harmful Deep Fakes and upholding freedom of expression rights, ensuring that legitimate use cases are not unnecessarily restricted.
The formulation of regulations requires collaboration between governments, tech companies, researchers, and civil society to address the ethical concerns surrounding Deep Fakes and harness their positive potential while mitigating the risks of misuse.
In the age of rapid technological advancements, Deep Fakes stand as a captivating yet perilous innovation. This article has embarked on a journey to explore the duality of Deep Fakes, questioning whether they are a threat to democracy or a tool for good. As we have delved into their positive contributions and insidious implications, one thing remains abundantly clear – the impact of Deep Fakes extends far beyond the realm of technology.
As we peer into this double-edged sword of innovation, we are confronted with the urgency of finding a delicate balance. Addressing ethical concerns, enacting robust regulations, and fostering technological advancements in detection are imperative steps to mitigate the risks of malicious Deep Fakes. Striking this balance is crucial to preserve the positive aspects of synthetic media while safeguarding the values that underpin democracy and human dignity.
It is incumbent upon governments, tech companies, researchers, and individuals alike to act responsibly and collaboratively in harnessing the potential of Deep Fakes for the greater good. Embracing media literacy, nurturing critical thinking, and cultivating healthy scepticism can empower individuals to discern between genuine and manipulated content.