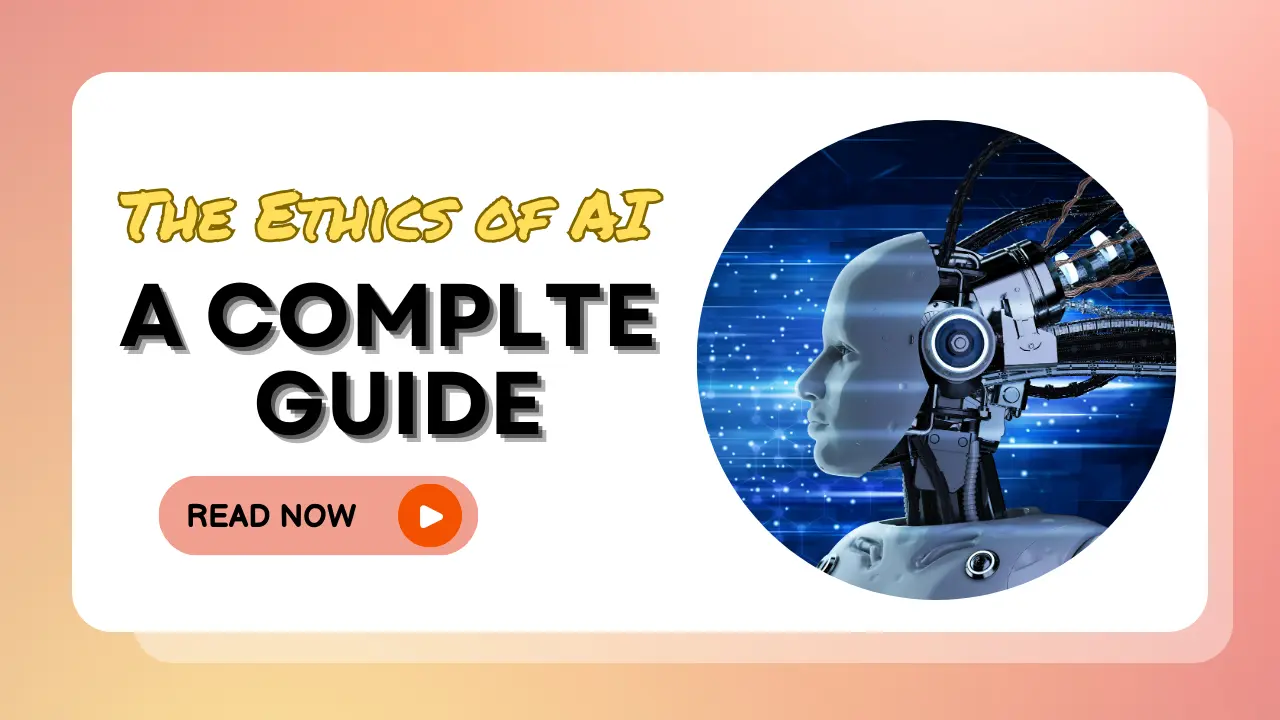
Index
Introduction
The Ethics of Artificial Intelligence: A Comprehensive Guide
In an era characterised by unprecedented advancements in technology, one field stands out as both a beacon of innovation and a moral minefield: Artificial Intelligence (AI). The rapid evolution of AI has brought forth a multitude of opportunities, from revolutionising healthcare and optimising industries to enhancing everyday conveniences. However, as we journey deeper into the digital age, we find ourselves standing at the crossroads of immense potential and profound ethical dilemmas. This comprehensive guide aims to illuminate the intricate landscape of AI ethics, offering a roadmap to navigate the complex moral challenges that arise in this brave new world of intelligent machines.
As AI systems continue to infiltrate various aspects of our lives, from autonomous vehicles and virtual assistants to algorithmic decision-making in criminal justice, healthcare, and finance, a pressing question emerges: How can we harness the transformative power of AI while ensuring that its development and deployment align with our shared values and principles?
In this guide, we will embark on a thought-provoking journey through the ethical dimensions of AI, exploring the intricate tapestry of issues that encompass privacy, bias, accountability, transparency, and more. We will delve into the philosophical foundations of AI ethics, tracing its historical roots and examining the profound ethical theories that underpin our discussions today.
Artificial Intelligence- What It Really Is
Artificial Intelligence (AI) refers to the simulation of human intelligence in computer systems and machines, allowing them to perform tasks that typically require human intelligence. This encompasses a range of capabilities, including problem-solving, learning, reasoning, natural language understanding, perception, and decision-making. AI systems are designed to analyse data, adapt to new information, and make autonomous decisions, often mimicking human cognitive functions.
Significance of AI in Modern Society
- Automation and Efficiency: AI has revolutionised industries by automating repetitive and labour-intensive tasks, leading to increased efficiency and cost savings. Manufacturing, logistics, customer service, and data analysis are some areas where AI-driven automation has made a significant impact.
- Healthcare Advancements: AI plays a crucial role in medical diagnosis, drug discovery, and personalised treatment plans. Machine learning algorithms can analyse vast datasets of patient information, aiding healthcare professionals in making accurate diagnoses and improving patient care.
- Enhanced User Experiences: AI powers virtual assistants like Siri and Alexa, chatbots, and recommendation systems that enhance user experiences in various domains, from e-commerce to entertainment. These systems understand and respond to natural language, making interactions more intuitive.
- Financial Services: In the financial sector, AI is employed for fraud detection, algorithmic trading, credit scoring, and risk management. It processes vast amounts of financial data in real time, helping institutions make informed decisions.
- Transportation and Autonomous Vehicles: AI is central to the development of self-driving cars, drones, and smart transportation systems. These technologies have the potential to improve road safety, reduce traffic congestion, and lower carbon emissions.
- Education: AI-powered educational platforms offer personalised learning experiences, adapting content and pacing to individual student needs. This can help bridge educational gaps and improve learning outcomes.
- Natural Language Processing: AI's ability to understand and generate human language has applications in translation, sentiment analysis, content generation, and more, facilitating global communication and content creation.
- Scientific Research: AI aids scientists in analysing complex data, predicting outcomes in experiments, and accelerating research in fields such as genomics, chemistry, and physics.
- Climate Change and Sustainability: AI assists in monitoring and managing environmental resources, such as predicting weather patterns, optimising energy consumption, and analysing climate data to combat climate change.
- Ethical and Societal Considerations: As AI becomes more integrated into society, ethical considerations related to bias, transparency, accountability, and privacy are of paramount importance. Ensuring responsible AI development and deployment is a significant challenge.
AI is a transformative technology with wide-ranging applications that have the potential to improve efficiency, drive innovation, and address complex societal challenges. Its significance lies in its ability to augment human capabilities, automate tasks, and make data-driven decisions, reshaping the way we live and work in the modern world. However, its responsible development and ethical use are essential to harness its benefits while mitigating potential risks.
History of AI
The history of Artificial Intelligence (AI) is a fascinating journey that spans several decades, marked by significant milestones and paradigm shifts in our understanding of machine intelligence.
Early Foundations (1940s-1950s)
The concept of AI can be traced back to the 1940s when mathematician and logician Alan Turing proposed the "Turing Test," a criterion for determining a machine's ability to exhibit human-like intelligence. In the mid-20th century, pioneers like John von Neumann and Norbert Wiener laid the groundwork for AI by developing theories related to computation and cybernetics. In 1950, computer scientist and logician Alan Turing introduced the idea of a "universal machine," which could simulate any other machine's behaviour through a series of instructions.
The Dartmouth Workshop (1956)
The term "Artificial Intelligence" was coined at the Dartmouth Workshop in 1956, where mathematician John McCarthy, along with Marvin Minsky, Nathaniel Rochester, and Claude Shannon, convened a group of researchers to explore the possibilities of creating intelligent machines. This event is often considered the birth of AI as an academic discipline.
Symbolic AI (1950s-1960s)
Early AI systems focused on symbolic reasoning and rule-based logic. Programs like the Logic Theorist and General Problem Solver aimed to solve problems through symbolic manipulation. The emphasis was on using formal rules and knowledge representation to simulate human thought processes.
AI Winter (1970s-1980s)
After initial enthusiasm, the field faced challenges, leading to periods known as "AI winters" when funding and interest waned due to unmet expectations and technical limitations. Critics argued that symbolic AI couldn't handle real-world complexities.
Rise of Expert Systems (1980s)
The 1980s saw a resurgence of AI research with the development of expert systems. These were AI programs designed to mimic human expertise in narrow domains, such as medical diagnosis and financial planning. Expert systems found practical applications in various industries.
Machine Learning and Neural Networks (1980s-1990s)
AI researchers began exploring machine learning techniques, including neural networks, during this period. Neural networks, inspired by the structure of the human brain, showed promise in pattern recognition and learning from data. The backpropagation algorithm, developed in the 1980s, helped train neural networks more effectively.
AI Renaissance (2000s-Present)
Advances in computational power and the availability of vast datasets have fueled the recent AI resurgence. Machine learning, especially deep learning, has led to breakthroughs in natural language processing, computer vision, and game-playing AI. Technologies like IBM's Watson, Google's DeepMind, and self-driving cars have captured the public imagination. AI applications have become ubiquitous in daily life, from virtual assistants like Siri to recommendation systems like Netflix.
Ethical and Societal Concerns
As AI becomes more pervasive, ethical and societal concerns have come to the forefront. Issues related to bias, transparency, accountability, and privacy are being actively addressed. Organisations and governments are developing guidelines and regulations to ensure the responsible development and deployment of AI.
The history of AI is characterised by periods of enthusiasm, stagnation, and resurgence. It has evolved from symbolic AI to machine learning, with modern AI systems leveraging massive datasets and powerful computing resources. The field continues to progress rapidly, promising transformative changes in how we live and work while posing significant ethical and regulatory challenges that require careful consideration.
Ethical Concerns Related to AI
Ethical concerns related to AI have grown in prominence as artificial intelligence technologies become increasingly integrated into various aspects of society. These concerns encompass a wide range of issues, including:
- Bias and Fairness: AI systems can inherit biases present in their training data, which can lead to discriminatory outcomes. For example, facial recognition software has exhibited racial and gender biases. Addressing bias and ensuring fairness in AI algorithms is crucial to prevent discrimination and promote equal treatment for all.
- Transparency and Explainability: Many AI algorithms, particularly deep learning models, are often seen as "black boxes" because their decision-making processes are not easily interpretable. Lack of transparency makes it difficult to understand how AI systems arrive at their conclusions, which can lead to mistrust and hinder accountability.
- Accountability: When AI systems make decisions that impact individuals' lives, it can be challenging to assign responsibility for errors or unintended consequences. Establishing clear lines of accountability is essential to address issues and ensure ethical behaviour in AI.
- Privacy: AI often involves collecting and analysing vast amounts of personal data. Unauthorised access or misuse of this data can violate individuals' privacy. Ethical concerns arise when AI systems are not adequately safeguarded against data breaches or unauthorised access.
- Job Displacement and Economic Impact: Automation driven by AI can lead to job displacement in certain industries, raising concerns about unemployment and economic inequality. Society must address the challenges of reskilling and ensuring that the benefits of AI are shared broadly.
- Autonomous Weapons and Lethal AI: The development of autonomous weapons, such as drones and robots, raises ethical questions about the use of AI in warfare and the potential for AI-driven lethal actions without human intervention. International efforts to regulate autonomous weapons are ongoing.
- Deepfakes and Misinformation: AI-generated deepfakes can convincingly manipulate audio, video, and text, making it challenging to discern real from fake content. Deep Fakes pose risks to personal and public trust, as well as the spread of misinformation.
- AI in Criminal Justice: The use of AI in criminal justice for risk assessment and sentencing decisions can perpetuate biases and discrimination if not carefully designed and monitored. Ethical concerns include fairness, transparency, and the potential for reinforcing existing inequalities.
- Surveillance and Social Control: The widespread deployment of AI-powered surveillance systems can lead to concerns about government and corporate control over individuals and erosion of civil liberties. Balancing security with privacy and freedom is a complex ethical challenge.
- Ethical Treatment of AI Systems: As AI becomes more sophisticated, questions arise about how we should ethically treat these systems. Some argue for granting AI systems certain rights or protections.
- Environmental Impact: The massive computational resources required for training deep learning models have raised concerns about the environmental impact, particularly in terms of energy consumption and carbon emissions.
Addressing these ethical concerns requires a multi-faceted approach involving researchers, policymakers, industry leaders, and society at large. Developing ethical guidelines, regulations, and best practices, along with fostering public awareness and engagement, are essential steps toward responsible AI development and deployment. Ethical considerations should be an integral part of AI development from its inception to ensure AI technologies benefit humanity while minimising harm.
Ethical AI Development
Ethical AI development involves adhering to principles and practices that ensure AI technologies are created and deployed in a responsible and ethical manner. Here are some best practices for ethical AI development:
- Data Quality and Bias Mitigation: Start with high-quality and diverse training data to reduce biases inherent in the data. Regularly audit and monitor datasets for biases and address them during training and testing phases. Consider the potential impact of bias on vulnerable or underrepresented groups.
- Transparency and Explainability: Strive to make AI models and decision-making processes transparent and interpretable. Use explainable AI techniques to provide insights into how AI systems arrive at their conclusions. Document the design, data sources, and evaluation methods comprehensively.
- Accountability and Responsibility: Clearly define roles and responsibilities within AI development teams, including who is responsible for addressing ethical concerns. Establish protocols for handling errors, unintended consequences, and ethical dilemmas that may arise.
- User Privacy and Consent: Prioritise user privacy and data protection by following relevant regulations (e.g., GDPR). Obtain informed consent when collecting and using personal data. Implement strong security measures to safeguard user data against breaches.
- Fairness and Anti-Discrimination: Continuously assess and mitigate biases in AI systems, particularly those that could lead to discrimination. Regularly audit AI systems to ensure they do not unfairly disadvantage any group or individual. Be transparent about fairness measures taken.
- Human Oversight and Control: Maintain human oversight in AI systems, especially in critical decision-making processes. Ensure that users have the ability to understand, challenge, and override AI decisions when necessary.
- Continual Monitoring and Evaluation: Regularly assess the performance of AI systems post-deployment to identify and rectify biases, errors, or unintended consequences. Monitor for ethical issues and adapt the system as needed over time.
- Ethical Guidelines and Frameworks: Adopt and adhere to established ethical guidelines and frameworks, such as the IEEE Ethically Aligned Design, to inform AI development and deployment. Seek external audits or ethical certifications when appropriate.
- Stakeholder Engagement: Engage with a diverse range of stakeholders, including ethicists, impacted communities, and the public, to gather input and perspectives on AI systems. Incorporate feedback into the development process.
- Education and Training: Train AI developers, engineers, and decision-makers in AI ethics and responsible AI practices. Foster a culture of ethical awareness and responsibility within AI development teams.
- Risk Assessment and Mitigation: Conduct thorough risk assessments to identify potential ethical issues and unintended consequences before deployment. Develop contingency plans for handling ethical challenges that may arise.
- Compliance with Regulations: Stay up-to-date with evolving legal and regulatory frameworks related to AI ethics, such as data protection and discrimination laws. Ensure compliance with relevant regulations in all phases of AI development and deployment.
- Ethical Impact Assessment: Implement an ethical impact assessment process to evaluate the potential ethical implications of AI projects before they begin.
- Public Communication and Transparency: Communicate openly with the public about the purpose, capabilities, and limitations of AI systems. Be transparent about the ethical principles and practices followed.
- Red Teaming and Ethical Hacking: Employ red teaming or ethical hacking to simulate potential misuse or ethical vulnerabilities in AI systems and address them proactively.
By integrating these best practices into the AI development lifecycle, organisations can create AI systems that not only deliver value but also adhere to ethical principles and promote trust among users and stakeholders. Ethical AI development is an ongoing process that requires vigilance, adaptability, and a commitment to upholding ethical standards throughout the technology's lifecycle.
As we conclude our exploration, it is abundantly clear that the ethical considerations surrounding AI are not mere abstractions. They are the touchstones that determine the direction of AI's impact on society, influencing our daily lives, industries, and the very fabric of our interconnected world. The decisions we make today about AI ethics will resonate for generations to come.
The future of AI lies in our collective hands, and our responsibility is two-fold: to harness the transformative power of AI to uplift humanity while vigilantly guarding against its potential pitfalls. It requires a commitment to transparent, accountable, and fair AI development practices. It demands ongoing dialogue among policymakers, technologists, ethicists, and citizens. It necessitates regulations and guidelines that align AI with our shared values.
As we embrace the boundless potential of artificial intelligence, let us remain steadfast in our dedication to ethical principles. Let us shape a future where AI is a force for good, where it empowers, enhances, and enriches the human experience, while always respecting the fundamental values of fairness, transparency, and accountability. The journey has only just begun, and it is incumbent upon us to ensure that AI's evolution remains a beacon of ethical excellence in an increasingly AI-driven world.